Mark III Systems' Introduction to Deep Learning: Session Two
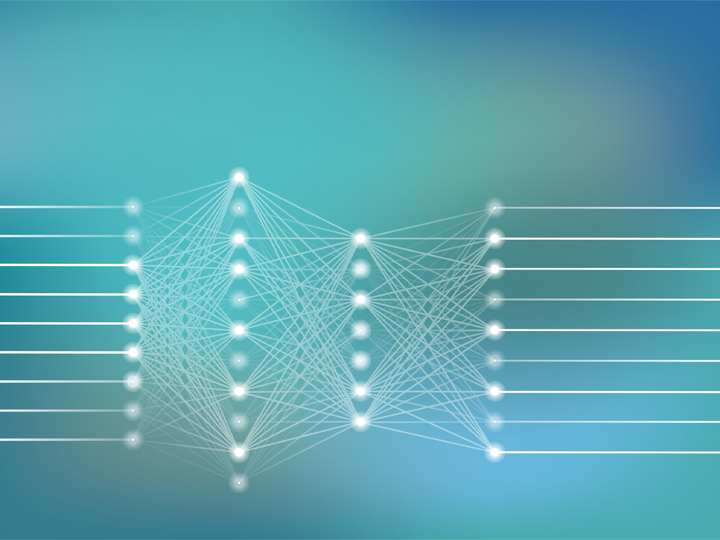
The webinar, produced in collaboration with the University of Houston’s HPE Data Science Institute, was conducted largely by Mark III’s Principal Data Scientist, Michaela Buchanan. She was introduced by Mark III’s VP of Strategy and Innovation, Andy Lin. Lin introduced Mark III Systems, stating that the solutions provider has “a strong practice and team in helping research organizations and enterprises build their AI, HPC, and Simulation Centers of Excellence.”
Buchanan led the session off with the acknowledgement that she would not be assuming any prior knowledge of DL for participants and that she would remain “more practical than academic.” Deep Learning, a subset of Machine Learning, which is within the circle of AI, is “the computation of multi-layer neural networks.” During Deep Learning, data scientists are identifying the type of algorithm that is being used. “This,” Buchanan stated, “is the neural network.” To illustrate her point, a biological neuron versus a model machine-drawn neuron were shown on the PowerPoint – and they resembled each other a great deal.
Inputting the data and changing weights and biases were the first steps to becoming familiar with a model with an accuracy as close to 100 percent as possible. Her example was training a program to distinguish a cat from a dog. Low resolution images were used for classification so as not to take up unneeded bandwidth. She discussed learning rates because if you go too small with a learning rate, you could be training a simple model for two weeks before getting your output!
Buchanan related the concept of “overfitting” which is sort of like when a teacher gives a practice test to a group of students – some will learn the answers and understand the material better, while others will merely memorize the practice test answers. That won’t really help them on the test proper. One way of overcoming overfitting is adding dropout layers in your neural network.
A tutorial finished up the webinar using CIFAR-10 and tf.keras, and questions were taken at the very end. The two-part series was a fantastic big picture view of how to maneuver within AI, ML and DL programming.